HEURISTICS
THE NEW AI FRONTIER - BALANCING HUMANITY AND AI
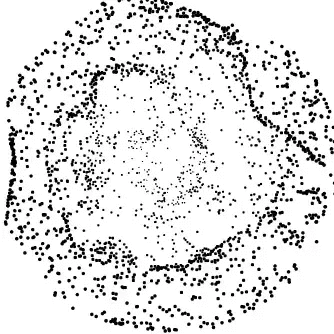
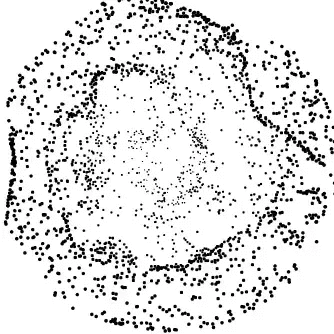
The word heuristic is derived from the Greek word “to discover.” You can think of heuristics as mental shortcuts that ease the cognitive load of making decisions. Examples that employ heuristics include using trial and error, a rule of thumb, an educated guess, an intuitive judgment, a guesstimate, profiling, or common sense. In their initial research, Tversky and Kahneman proposed three heuristics—availability, representativeness, and anchoring and adjustment. Subsequent work has identified many more.
Heuristics that underlie judgment are called "judgment heuristics." If you want to look at it from a more personal perspective, a heuristic is a mental shortcut that allows people to solve problems and make judgments quickly and efficiently. These rule-of-thumb strategies shorten decision-making time and allow people to function without constantly stopping to think about their next “perfect” course of action.
The accuracy-effort trade-off theory states that humans and animals use heuristics because processing every piece of information that comes into the brain takes time and effort. With heuristics, the brain can make faster and more efficient decisions, albeit at the cost of accuracy. A heuristic, heuristic rule, or heuristic program is a common-sense rule (or set of rules) intended to increase the probability of solving some problem. Cognitive psychology recognizes many types of heuristics that are part of human knowledge and decision-making processes.
A classic example is the Substitution Heuristic, which causes a person to substitute a complex question with a simpler one to provide an initial answer. For example, imagine an oncologist examining a cancer patient trying to answer a question such as: How likely is this tumor to metastasize? can come up with a probability by answering the heuristic question: How sick does the patient look today? The heuristic question-answer is not based on a detailed evaluation of the patient’s symptoms but rather on the subjective opinion of the physician. Another example of heuristic is known in psychology as the recognition heuristic, which states that we tend to associate a hypothesis with a subject if we recognize it. For instance, a subject asked which of two (relatively big) cities is larger and recognizes one of them should guess that the one he/she recognizes is the largest.
One of my favorite heuristics is what is known in economics as the Law of Small Numbers, which states that many conclusions in experiments have been determined without sampling a large enough data set. To validate an experiment, experts often choose samples simply too small to arrive at a meaningful conclusion, but that doesn’t preclude them from asserting the results as valid. In the business world, sometimes this materializes as people who make decisions based on a single event or so few data points it would be impossible to make an informed decision. Still, in the interest of time, they rely on imperfect information to move forward. There are many other well-known heuristics in the cognitive psychology space, such as Confidence-Doubt or Cause-Chance.
Over the years, heuristics have stamped their fingerprint over human knowledge and data. Heuristic analysis is also a method of detecting viruses by examining code for suspicious properties. Most common today, heuristic analysis is incorporated into advanced security solutions offered by many high-tech companies to detect new threats before they cause harm without the need for a specific signature. Now, the same heuristic-based knowledge is becoming the source of information we use to train AI agents. As a result, many AI systems reflect cognitive patterns that are highly illogical and have no correlation to statistical analysis principles. Here’s where using heuristic-based techniques gets weird. Take heuristic-based thinking in AI agents as an example. For starters, the experts in charge of training AI systems are humans and, therefore, vulnerable to heuristic analysis, making for an ironic vicious circle.
When you dig deeper, this approach to decision-making almost mimics our imperfect human condition, creating the perfect scenario to transform a binary decision-making engine grounded in math into one that makes decisions based on its best guess or “gut feeling” – the feared too human-like AI with strong binary math driven analysis capabilities driven by flexible heuristic based learning and decision making.
Super creepy, right?